What Is Data Intelligence? Overview, Types & Benefits
Contact Us | |
Free Demo | |
Chat | |
Leveraging data intelligence can help optimize performance and give your organization a competitive advantage. In this blog we look at other benefits, how it works, and the many different types of data intelligence.
In this information age, mentioning data and intelligence in the same sentence almost seems redundant. This is because copious amounts of data on your industry, customers, and competitors don’t translate to a competitive advantage.
Good corporate decision-making is underpinned by accurate data intelligence that makes sense of the voluminous realms of data generated daily from websites, social media, and IoT sensors.
What Is Data Intelligence?
Data intelligence refers to using advanced systems and methods to analyze, understand, and leverage large volumes of data for strategic decision-making. It involves collecting and processing data, extracting insights, and using these insights for predictive and prescriptive purposes.
Why Does Data Intelligence Matter?
Data intelligence can provide crucial information about market trends, customer behavior, operational efficiency, etc. This helps organizations to optimize performance, make informed decisions, and create competitive advantages.
Data intelligence often uses technologies like artificial intelligence and machine learning to automate and improve the analysis process.
- Informed Decision Making: Data intelligence processes large amounts of information and provides insights that help businesses make data-backed decisions, including better protecting sensitive information.
- Competitive Advantage: It can provide businesses with a competitive edge by identifying market trends, customer preferences, and potential business growth areas that might not be visible otherwise.
- Predictive Analysis: Data intelligence tools enable business leaders to forecast future trends, helping them prepare for opportunities and mitigate challenges.
- Risk Management: From identifying potential fraud patterns to ensuring regulatory compliance, data intelligence can help minimize various business risks.
- Personalized Experiences: With a better understanding of customer habits and preferences, businesses can tailor their products, services, and interactions to deliver personalized customer experiences.
- Enhanced Product Development: By understanding market trends and consumer demands, businesses can improve their product development processes, creating products and services that meet the needs of their customers more effectively.
- Better Return on Investment: Businesses can make more strategic decisions about where to invest their funds and get a higher return on investment.
- Data Governance: Data intelligence can support data governance, managing and ensuring the quality, integrity, and security of the data. This is particularly important in a world where data privacy regulations are becoming increasingly stringent.
What Are the Benefits of Data Intelligence?
- Informed Decision-Making: Data intelligence provides valuable insights that can guide strategy and decision-making, leading to better outcomes and improved competitiveness.
- Enhanced Customer Understanding: It can help businesses understand their customers’ behaviors and preferences, enabling targeted marketing and improved customer service.
- Improved Efficiency: Data intelligence can highlight areas for improvement within a business, leading to streamlined operations and increased productivity.
- Risk Management: By identifying patterns and trends, data intelligence can predict potential risks or issues before they occur, enabling businesses to mitigate them proactively.
- Predictive Insights: With the help of machine learning algorithms, data intelligence can provide predictive insights about future outcomes, which can be a valuable tool for strategic planning.
- Personalization: Data intelligence can allow businesses to offer personalized experiences to their customers, leading to increased satisfaction and loyalty.
- Innovation: The insights derived from data intelligence can fuel innovation, leading to new products, services, or business models.
- Compliance: Data intelligence tools can help businesses comply with regulatory requirements by ensuring their data is accurate, complete, and secure.
Data Intelligence Use Cases and Examples
- Predictive Analytics: Companies can use previous data to predict future outcomes. For example, utilizing customer purchase history and browsing data to anticipate future buying behavior. They can also provide actionable threat intelligence to thwart potential attacks.
- Customer Segmentation: Using customer data analytics, businesses can determine various customer segments to tailor their marketing strategies accordingly.
- Product Recommendations: Online retailers can use past purchase data and browsing habits to suggest relevant products to customers.
- Fraud Detection: In the banking and finance industry, data intelligence can detect unusual transactions or activities that may indicate fraud.
- Supply Chain Optimization: Companies can analyze historical shipment data and real-time transport conditions to optimize delivery routes and times, improving efficiency and cost-effectiveness.
- Healthcare Diagnosis and Treatment: Data intelligence can aid in diagnosing diseases and suggesting personalized treatment plans by analyzing patient data, medical history, and recent advances in medical research.
- Social Media Sentiment Analysis: Companies can gauge public opinion and sentiment about their brand or product by analyzing social media data.
- Maintenance Prediction: In industries with heavy machinery, predictive analytics can anticipate when a machine might break down, enabling preventive maintenance.
What are the Types of Data Intelligence?
Several types of data intelligence exist, including:
- Predictive Analytics: Predictive analytics looks forward, predicting potential events and patterns based on existing data.
- Prescriptive Analytics: The intelligence prescribes or suggests operationally viable recommendations and action strategies based on analysis of different data scenarios.
- Descriptive Analytics: Descriptive analytics focuses on understanding and describing historical data, offering insights into what has occurred.
- Diagnostic Analytics: Diagnostic analytics considers why something occurred and identifies trends and correlations in datasets.
- Behavioral Analytics: Behavioral analytics involves deeply analyzing consumer behavior to predict future actions and habits.
- Qualitative Analytics: Qualitative analytics primarily involves the analysis of non-numerical data such as text, images, audio, and video.
- Quantitative Analytics: This form of intelligence involves the analysis of numerical data that can be quantified, such as metrics and statistics.
- Competitive Intelligence: Competitive intelligence analyzes the competition's data to strategize and make informed decisions.
- Machine Learning Analytics: These algorithms analyze data to find trends, develop predictions, and learn from results over time, enhancing their accuracy.
- Real-Time Analytics: As the name suggests, real-time analytics analyzes data as it comes in, providing insights instantaneously or close to real-time.
How Does Data Intelligence Work?
Data intelligence works by conducting a systematic analysis of an organization's data. Here is a generalized overview of how the process works:
- Data Collection: The first step in data intelligence is gathering data from various sources. This could include data from internal systems such as CRMs, ERPs, transaction history, social media, websites, etc.
- Data Cleaning: Once data is collected, it has to be cleaned to eliminate duplicates, correct errors, and handle missing information. Clean data is crucial for accurate analysis.
- Data Integration: The cleaned data from different sources must be integrated and consolidated for comprehensive analysis. This process could involve complex matching of fields from disparate data sources.
- Data Analysis: Using different data analytics methods and tools, data are analyzed to uncover patterns, correlations, and trends. Techniques can vary from statistical analysis to machine learning and artificial intelligence methods.
- Data Visualization: The analyzed data is then visualized using charts, graphs, and other visual tools. This helps in presenting the interpreted data in an easily understandable format.
- Generation of Insights: The final stage involves generating actionable insights from the patterns and relationships identified in the data. These insights can directly influence strategic decisions and operations within the organization.
- Application of Insights: The generated insights are then applied towards improving business processes, identifying potential market trends, predicting customer behavior, optimizing resource allocation, and more.
What Are the Technologies that Support Data Intelligence?
Several technologies support data intelligence, including:
- Artificial Intelligence (AI): AI can automatically analyze and interpret patterns in big data. It can also help predict future trends based on historical data.
- Machine Learning (ML): ML, a subset of AI, automates building analytical models. It uses algorithms to parse data, learn from it, and then make decisions or predictions about something in the world.
- Big Data Analytics: Insightful data discovery fueled by powerful software and algorithms examines large amounts of data and uncovers hidden patterns, correlations, and other insights.
- Data Warehousing: This is a large store of data collected from various sources within a company to guide management decisions. It helps in data analysis and reporting.
- Data Mining: Companies use the process of turning raw data into useful information by discovering patterns in large data sets.
- Blockchain technology: It can be used to maintain transparency and ensure data integrity and security.
- Cloud Computing: The cloud provides a platform to access, store, manage, and process data. It offers scalable and flexible solutions for data storage and analysis.
- Internet of Things (IoT): IoT devices capture and transfer data, contributing to the generation of massive data sets that can then be analyzed for insights.
- Natural Language Processing (NLP): NLP helps analyze text data, understand its sentiment, and extract useful insights.
- Robotic Process Automation (RPA): RPA allows organizations to automate routine and repetitive tasks, which can help streamline data collection and processing.
- Data Visualization Tools: These software present data in a pictorial or graphical format, enabling businesses to identify patterns, trends, and insights in data.
How Do You Implement Data Intelligence?
Implementing data intelligence in an organization involves several steps:
- Define Goals and Objectives: Clearly define what you want to achieve with data intelligence. This could range from improving decision-making, predicting customer trends, enhancing operational efficiency, or complying with regulations.
- Identify Relevant Data: Identify the data you need for your objectives. This could be customer, financial, operational, or even unstructured data from social media or other sources.
- Data Collection and Integration: Collect relevant data from disparate sources and integrate it into a unified platform. This can involve using data integration tools.
- Data Cleaning and Preparation: Once data is collected, it must be cleaned and prepared for analysis. This involves removing duplicates, dealing with missing values, and ensuring data is in the right format.
- Data Analysis and Interpretation: Use advanced analytics techniques like machine learning or artificial intelligence to analyze the data and extract insights.
- Implement a Data Governance Framework: This includes setting data quality standards, managing data access and security, and maintaining metadata.
- Visualize Insights: Use data visualization tools to showcase insights in an easily digestible format so they can inform strategic decision-making.
- Continually Monitor and Update: Data intelligence is not a one-time process – data should be continually monitored, and the analytics models updated as needed.
- Train Employees: Lastly, but very importantly, employees must be trained to interpret and utilize the insights generated. A culture of data literacy should be encouraged.
The Benefits of Partnering with a Data Intelligence Company Like Digital Guardian
Digital Guardian has the tools, experience, and expertise to help you make sense of your data, whether voluminous, structured, or unstructured, for improved business performance.
Our vast data scanning capabilities provide scalability and flexibility to protect intellectual property (IP), whether at rest or in transit.
Schedule a demo with us today to learn how to boost your data intelligence.
Recommended Resources

All the essential information you need about DLP in one eBook.

Expert views on the challenges of today & tomorrow.
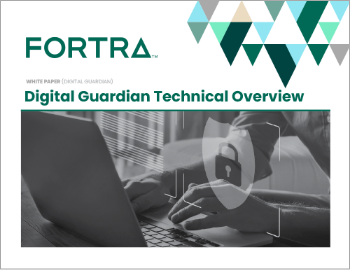
The details on our platform architecture, how it works, and your deployment options.
Don't Fall Behind
Get the latest security insights
delivered to your inbox each week.
Thank you for subscribing!