Building a Strong Foundation: 6 Takeaways from Forrester’s Rethinking Data Discovery & Classification Report
Contact Us | |
Free Demo | |
Chat | |
Forrester's report on data discovery & classification has several key takeaways for any data loss prevention program. I'd like to share my personal favorites.
In an earlier post, I discussed key items from Forrester’s report, The Future of Data Security: A Zero Trust Approach. That report focused on the problem with perimeter defenses in a world where the perimeters are disappearing. Their second report in the series - Rethinking Data Discovery and Data Classification – addresses the task of identifying sensitive data in an organization. Forrester makes a strong argument for building a repeatable process for defining, analyzing, and defending data, with some valuable advice on how to do so. Here are my takeaways:
1. Data classification is fundamental to any security program
While it’s important to recognize that data classification is integral to an effective DLP initiative, it’s not simply a DLP-related effort or a form of DLP. Classification is the foundation for all data security, including DLP.
To protect information, the target of most attacks, you need to know which data is critical, where it resides, and how it is used. Aside from pure DLP efforts, this information will help determine which assets to prioritized for hardening, redundancy, and testing.
Any security program requires prioritization. From a network standpoint, this typically starts with Internet-facing devices, as those are most accessible to attackers. Data prioritization can seem more difficult, as it can reside inside or outside the enterprise. Protecting this data is a primary goal of any security program, making data classification critical, not simply for DLP.
2. Start at the beginning.
“For many S&R pros, data security initiatives quickly zoom in on controlling access to data, or encrypting data. What many overlook is that understanding and knowing your data is the foundation for data security.”
The goal of data protection is prevent misuse of data, while allowing unfettered legitimate use. The “simple” path used by some organizations is to encrypt all data, relieving the organizations of determining which information is and isn’t important. Similarly, fine-grained access control can prevent unauthorized users from viewing data.
It surprises many security professionals that this is actually a more complicated approach to data loss protection. Do you really know every person, inside and outside your organization, who requires access to every document? How do you address the malicious insider? Making a mistake with access control can result in either allowing access to unauthorized users or blocking a legitimate user from doing her job.
Defining what data is sensitive, and how sensitive, is a critical first step in any information security program. A data-centric approach focuses on an organization’s most critical information, and enforces controls not only on who can use the data, but under which circumstances. This can mean that data can be accessed locally, but not off the network. It can be moved to company-approved removable drives, but forces encryption of the sensitive data. Focusing on the data that is most critical to your organization simplifies security efforts.
3. Don’t boil the ocean
“A typical enterprise data classification scheme has anywhere from three to six levels. Forrester has seen organizations with as many as nine levels of classification. The complexity involved with identifying unique criteria for so many levels — and then having employees understand the difference and apply labels correctly — is a ticking time bomb waiting to explode.” Other dimensions such as likelihood and impact of a data breach may be taken into consideration.
Once data is identified, it may be appealing to apply sophisticated classifications and controls to everything. Most successful programs, however, are iterative. By starting with data classification, organizations have the advantage of perspective; they have identified their critical data and can more easily prioritize their DLP efforts to focus on what Forrester refers to as the most “toxic” data.
Start with the data you consider your core IP and apply simple classifications. This may be design information for manufacturing companies, PHI for healthcare companies, or credit card information for financial services and retailers. In each case, all of this information is critical and sensitive. Monitor data use to determine how it may be put at risk, and then build controls to mitigate that risk. Once this program is running, move to the next critical data category. Starting small is easier to manage and more likely to succeed.
4. Your employees want to help, but you shouldn’t make them do so
The traditional solution in a data classification project rollout is to educate employees about the different classification levels, their respective markings, and when to apply them. The challenge here is that if these levels are not clear-cut and easily discernible, data classification becomes subjective and opportunistic. Employees don’t have time — or much desire — to parse through multiple levels of classification labels; they just want to do their job.
Manual classification of data is more time consuming and less consistent than automated classification. Classifying data by content inspection (e.g., looking for patterns indicating social security or credit card numbers) or contextual awareness (e.g., classifying data based on the application, author, or storage location) simplifies a DLP program. It does so without forcing employees to learn new skills, or interrupting their current workflow.
Similarly, by applying classification “tags” to data and enforcing policies on the endpoint, you can use “prompts” to remind users when an action may put data at risk. This provides data security training in real-time, allowing users to self-correct. We know that continuous training produces better results compared to scheduled training sessions. Your DLP program can provide this training without interfering with permitted activities.
5. Classifications aren’t written in stone
“The issue with data classification for new data is enforcement and how to address changes in classification when the need arises.” … ”Treat data as living, not static. Its value is highest at the point of creation, and over time may diminish.”
Data isn’t static, classifications shouldn’t be either. Financial information is released publicly, marketing plans are executed, and new products are released. A word document with social security numbers should be classified as sensitive. When those numbers are removed from the document, however, the classification no longer applies. Conversely, if those social security numbers are copied to a new document, it should be reflected in the classification of that document.
This call for intelligence at the endpoint, where toxic data is added or removed. Content inspection classification accomplishes this without forcing users to remember to classify the document manually. In addition, “inheritance” can apply the classification of a source document to any derivative document.
6. Think like an attacker
There are only two types of data that exist in your organization: 1) data that someone wants to steal and 2) everything else.
A consistent theme in Forrester’s report is that organizations are well served by simplification. You don’t need to start with a dozen data classifications covering every piece of data in your organization. Instead, think like an attacker to identify the data most attractive to an adversary. Then, use a method that automatically classifies that data as it is created. Finally, put controls on the endpoints to monitor and block actions that put that data at risk.
Starting a successful DLP program doesn’t have to be complicated. Organizations need to follow a structured process to identify their most sensitive data, classify it appropriately and monitor its use on endpoints. It needs to be flexible to accommodate changes in the environment and corresponding changes to the sensitivity of the data. Successful programs are also iterative, starting small and building on success.
Recommended Resources

All the essential information you need about DLP in one eBook.

Expert views on the challenges of today & tomorrow.
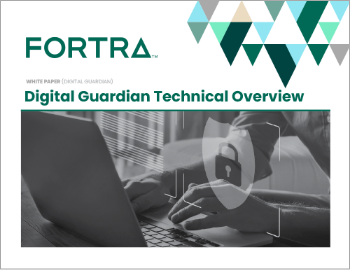
The details on our platform architecture, how it works, and your deployment options.