What Is Data Discovery? Process & Best Practices
Contact Us | |
Free Demo | |
Chat | |
Data discovery should be a cross-function effort for organizations. Read this blog to learn some of the processes needed to help facilitate data discovery, along with recommended best practices.
The proliferation of data as a valued resource in the digital age has placed a high premium on data discovery. Without effective data discovery, organizations cannot leverage and maximize the potential of their digital assets.
What Is Data Discovery?
Data discovery is a process developed to make it easier for stakeholders to find and identify data, including recognizing its patterns and trends. This process often uses visual tools and applications to extract insights, which can be used to drive decision-making within an organization.
The aim of data discovery is to turn data into valuable, actionable business insights. Data discovery often works in tandem with data classification since the latter assists in sorting and prioritizing data based on its sensitivity and vulnerability.
Why Data Discovery Is Important For Organizations
While locating critical data is important, data discovery goes beyond this. Data discovery can also be construed as a business-oriented data science process that yields analytical insight and detects patterns.
Here are some of the advantages and valuable insights that data discovery bestows:
- Informed Decision-Making: It enables businesses to gain insights from large datasets and make informed decisions. Without data discovery, important patterns and trends might go unnoticed.
- Enhanced Business Intelligence: Data discovery can enhance business intelligence by identifying key insights that provide a more comprehensive understanding of performance metrics and operations.
- Improve Efficiency: By identifying patterns and trends, businesses can streamline their operations, improve efficiency, and cut costs.
- Risk Management: It can help identify and mitigate potential risks before they become problematic. This can include everything from financial risk to operational risk.
- Compliance: Businesses that comply with regulations such as GDPR or HIPAA need to know their data, where it is, and who has access to it. Data discovery can assist in achieving these compliance standards.
- Customer Insight: Understanding customer behavior is critical to business success. Data discovery can reveal patterns in customer behavior that can be used to improve products and services and ultimately increase customer satisfaction.
- Innovate and Maintain Competitive Advantage: The insights derived from data discovery can identify opportunities for innovation, keeping businesses competitive in their respective industries.
Data Discovery Use Cases
As a subset of business intelligence, here are some of the uses and applications to which data discovery is applied:
- Customer Analytics: Companies can use data discovery to analyze customer behavior and trends, informing personalized marketing campaigns, product development, and other customer-related strategies.
- Fraud Detection: Data discovery can help identify patterns and anomalies that suggest fraudulent activity. This is particularly important in sectors like finance, insurance, and cybersecurity.
- Operational Efficiency: Businesses can use data discovery to analyze operational data, helping to identify inefficiencies, bottlenecks, or areas for improvement in workflows or processes.
- Risk Management: By identifying trends and patterns in data, businesses can better predict and mitigate risks, helping them to make more informed strategic decisions.
- Market Research: Data discovery can be crucial in understanding market trends, competitor activities, and changing customer preferences. This can better inform business planning and strategy.
- Supply Chain Optimization: Analyzing data from different points in a supply chain can lead to improved demand forecasting, inventory management, and logistics planning.
- Enhancing Productivity: Data discovery can help identify patterns and correlations in workforce data, which can be used to enhance staff productivity and improve workplace satisfaction.
- Compliance and Governance: For companies that must comply with regulations such as GDPR, data discovery can help identify and classify data, enabling them to manage and protect it properly.
- Personalizing User Experience: In the digital product space, data about how users interact with a platform can lead to personalization and improved user experience.
What Are the Types of Data Discovery?
There are three types of data discovery, namely visual, guided, and self-service, and enumerated below are each of their different purposes:
- Visual Data Discovery: This type of data discovery involves using various visual exploration tools to represent data in charts, graphs, and other visual forms. It aids in identifying patterns, correlations, and trends in data that may not be immediately apparent in raw, numerical data.
- Guided Advanced Analytics: This category uses more advanced statistical and predictive techniques to guide users through the data discovery process. These tools usually involve machine learning or AI capabilities, which can help identify deeper trends, make predictions, and provide insights.
- Self-Service Data Preparation: This type of data discovery allows end-users to access, integrate, cleanse, and prepare data for analysis. This can greatly increase efficiency within an organization because users do not need to rely on IT or data professionals to prepare data for them.
The Step-by-Step Processes that Facilitate Data Discovery
Data discovery involves a series of steps to gather and analyze data for useful insights, such as the following:
- Identify Needs and Set Goals: The first step of any data discovery process is identifying the business needs and setting clear objectives. These goals will guide the type of data that needs to be discovered and analyzed.
- Data Collection: The next step involves collecting data from diverse sources, including databases, documents, spreadsheets, cloud storage, social media feeds, and more.
- Data Integration: Once the required data is collected, the next step is to integrate this data on a common platform to facilitate efficient data, exploration, and analysis.
- Data Cleaning and Preprocessing: In this step, data scientists clean and preprocess the data to eliminate inconsistencies or errors, fill missing values, or remove duplicates. This provides quality and accurate data for analysis.
- Data Exploration: During this phase, analysts use statistical frameworks and visualization tools to explore data, summarizing its main characteristics and making it easier to draw insights.
- Modeling and Analysis: Analysis is used to identify patterns and relationships between different data elements using various algorithms and statistical methods.
- Interpretation and Insight Generation: The findings from the data analysis are then interpreted to generate insights that can answer the business questions identified in the first step.
- Reporting: The last step involves creating reports or dashboards that effectively communicate these insights to key stakeholders for data-driven decision-making.
- Iterate and Refine: This is a continuous process where analysts iterate and refine the process as new data or business needs change.
What Are the Data Discovery Best Practices?
- Use Suitable Tools: Different types of data require different discovery processes, often requiring different tools. Businesses should employ the right combination of tools that align with their specific data and analytical needs.
- Prioritize Data Governance: Implementing a robust data governance framework ensures accurate, consistent, and secure data. Data governance also allows businesses to establish rules and policies for data usage, ensuring everyone involved in the data discovery process understands their roles and responsibilities.
- Collaborative Approach: Data discovery should be a cross-function effort with collaboration among business analysts, data scientists, IT professionals, and decision-makers. This collective effort ensures data insights align with business goals and strategies.
- Training and Literacy: Continuously improving and updating the skills and knowledge of the business users engaged in data discovery is essential. This includes training on data discovery tools, understanding data interpretation, and making data-driven decisions.
- Regular Data Audits: Regularly conducting audits of the data being used in discovery processes allows businesses to ascertain the correctness and relevance of their data. This ensures that only the most valuable insights are unearthed and applied to real business situations.
- Data Security: It is essential to have strong data security measures, including encryption and access controls, to protect sensitive and confidential information.
- Continuous Learning and Improvement: The insights derived from data discovery should constantly be utilized to improve business strategies and decision-making processes.
- Value Measuring: Businesses must find ways to measure the value that data discovery provides, whether that is in improving decision-making, identifying opportunities, or reducing risks and costs.
- Opt for Automated Process: In the age of huge volumes of data and advanced analytics, automation can be a key factor in making data discovery more efficient and effective. Automated data discovery tools use machine learning and AI to speed up the process, automate repetitive tasks, and deliver deeper insights.
Digital Guardian Can Help With Your Data Discovery Process
Digital Guardian uses its innovation, like configurable scanning, to let you find data smartly, maintain visibility, and protect sensitive data at rest.
Schedule a demo today to learn more about how we can help organizations with their data discovery process.
Recommended Resources

All the essential information you need about DLP in one eBook.

Expert views on the challenges of today & tomorrow.
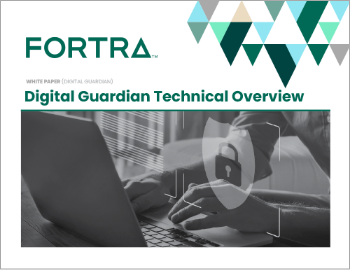
The details on our platform architecture, how it works, and your deployment options.
Don't Fall Behind
Get the latest security insights
delivered to your inbox each week.
Thank you for subscribing!